TESTIMONY OF JENNIFER PAHLKA, FORMER US DEPUTY CHIEF TECHNOLOGY OFFICER, SENIOR FELLOW, FEDERATION OF AMERICAN SCIENTISTS AND THE NISKANEN CENTER
BEFORE THE COMMITTEE ON HOMELAND SECURITY AND GOVERNMENT AFFAIRS, U.S. SENATE
ON HARNESSING AI TO IMPROVE GOVERNMENT SERVICES AND CUSTOMER EXPERIENCE
Chair Peters, Ranking Member Paul, and members of the Committee, I appreciate you inviting me here today to speak on this critical topic.
How the US government chooses to respond to the changes AI brings is indeed critical, especially in its use to improve government services and customer experience. If the change is going to be for the better (and we can’t afford otherwise) it will not be primarily because of how much or how little we constrain AI’s use. Constraints are an important conversation, and AI safety experts are better suited to discuss these than me. But we could constrain agencies significantly and still get exactly the bad outcomes that those arguing for risk mitigation want to avoid. We could instead direct agencies to dive headlong into AI solutions, and still fail to get the benefit that the optimists expect. The difference will come down to how much or how little capacity and competency we have to deploy these technologies thoughtfully.
There are really two ways to build capacity: having more of the right people doing the right things (including but not limited to leveraging technology like AI) and safely reducing the burdens we place on those people. AI, of course, could help reduce those burdens, but not without the workforce we need – one that understands the systems we have today, the policy goals we have set, and the technology we are bringing to bear to achieve those goals. Our biggest priority as a government should be building that capacity, working both sides of that equation (more people, less burden.)
Building that capacity will require bodies like the US Senate to use a wide range of the tools at its disposal to shape our future, and use them in a specific way. Those tools can be used to create mandates and controls on the institutions that deliver for the American people, adding more rules and processes for administrative agencies and others to comply with. Or they can be used to enable these institutions to develop the capacity they so desperately need and to use their judgment in the service of agreed-upon goals, often by asking what mandates and controls might be removed, rather than added. This critical AI moment calls for enablement.
The recent executive order on AI already provides some new controls and safeguards. The order strikes a reasonable balance between encouragement and caution, but I worry that some of its guidance will be applied inappropriately. For example, some government agencies have long been using AI for day to day functions like handwriting recognition on envelopes or improved search to retrieve evidence more easily, and agencies may now subject these benign, low-risk uses to red tape based on the order. Caution is merited in some places, and dangerous in others, where we risk moving backwards, not forward. What we need to navigate these frameworks of safeguard and control are people in agencies who can tell the difference, and who have the authority to act accordingly.
Moreover, in many areas of government service delivery, the status quo is frankly not worth protecting. We understandably want to make sure, for instance, that applicants for government benefits aren’t unfairly denied because of bias in algorithms. The reality is that, to take just one benefit, one in six determinations of eligibility for SNAP is substantively incorrect today. If you count procedural errors, the rate is 44%. Worse are the applications and adjudications that haven’t been decided at all, the ones sitting in backlogs, causing enormous distress to the public and wasting taxpayer dollars. Poor application of AI in these contexts could indeed make a bad situation worse, but for people who are fed up and just want someone to get back to them about their tax return, their unemployment insurance check, or even their company’s permit to build infrastructure, something has to change. We may be able to make progress by applying AI, but not if we double down on the remedies that failed in the Internet Age and hope they somehow work in the age of AI. We must finally commit to the hard work of building digital capacity.
History of digital enablement of services in government
Customer experience changed dramatically during the Internet era – we no longer wait in line at the bank to deposit a check or at the airport for a taxi. Many of the interactions we used to think of as customer service have disappeared, submerged into a layer of technology and data that answers the questions customer service used to ask. Who are you? Your bank knows. Where are you? Your ride hailing service knows. The public mostly likes these changes, but more importantly, it expects them. It now feels odd, even a little scary, to be asked questions the institution should know the answer to. It’s hugely frustrating to wait weeks, even months, for an answer that you know relies on some basic math a computer could do in nanoseconds if it just were just allowed to process the data you have just given it. “This isn’t that hard,” the veteran says as his application languishes in a backlog. We’ve made a lot of progress, but we are still struggling to gain the benefits the Internet era offered, (the White House recently wrote that only two percent of government forms are available online!),1 and the next era is already upon us.
How did we get here? A little history helps explain. Starting as far back as the 1960s, but particularly in the 1990s, when companies like Amazon and Google were emerging, leadership in government (both Democrats and Republicans) mistook what ultimately proved to be a massive digital revolution for a mere tactical shift in the tools of implementation. Tools are things you buy, so leadership saw digital as a problem of purchasing. Instead of recognizing that no institution, public or private, would be able to operate effectively in the coming decades without basic digital competence, and therefore hiring people who understood this brave new world, our government developed extensive processes and procedures for buying digital technology as if it were simply a commodity. Today, as we bemoan the lack of expertise in highly specialized, complex domains like advanced software, it’s worth noting that the inner workings of procurement seem as specialized, complex, and mysterious to the layperson as the inner workings of an AI model. Government is clearly capable of developing capacity in specialized domains. We just picked the wrong ones.
We have treated digital much like we treat pens, paper clips, or vehicles that the General Services Administration buys for agencies: we don’t need to know how it works, we just need to acquire it. Once we’ve acquired it, other than perhaps a maintenance contract, we’re done. Today, though it takes us a painfully long time to do so, government knows how to acquire static software. What we need to acquire are capabilities.
Flexible capabilities
Like most of what I will cover today, buying static software like we buy pens or cars was not a good idea in the Internet era, but it is a catastrophically bad one in the AI era. Software systems were always less static than our procurement frameworks allow for, and AI is orders of magnitude more dynamic. AI systems have all the dynamic characteristics of the previous software era, but are literally learning all the time, and therefore constantly changing in ways that we don’t entirely understand. Therefore, responsible and effective use of AI must involve constant learning and testing in the real world. Academics have shown, for instance, that an AI system developed on one university’s hospital patient data can perform radically differently if deployed to a different hospital setting or as patient profiles change over time.2 Our current “once and done” frameworks don’t allow for this ongoing evaluation, and our workforce is not suited to these challenges. We cannot simply engage procurement officers to evaluate and purchase a system like that, and hope it works out. AI demands agility and competence in ways we can no longer afford to ignore.
To illustrate the limitations of our legacy government procurement frameworks, it might be helpful to hear an example of what it’s been like for government technologists trying to guide a previous transition: the move to the cloud. One of the early recruits to 18F, Jez Humble, was working with a contracting officer in an attempt to purchase cloud services. Jez had prepared enormous amounts of data in advance of meeting with the contracting officer, but in the meeting, he found that he lacked the one piece of information the officer needed: how much these cloud services would be used. The officer could not put out a bid to procure a service if he didn’t know how much he would be asking for.
One of the key advantages of cloud computing, of course, (though not the only one) is the flexibility it offers. Instead of having to guess how much infrastructure you’ll need well ahead of launching, say, a website, and buying what you hope is the right number of servers and the sufficient bandwidth, you can essentially rent a flexible amount of capacity from a cloud computing provider and only pay for what you use. If traffic is less than expected, you save money. If it’s more, you pay more, but at least your website stays up as the cloud provider seamlessly handles the extra load. Jez couldn’t tell the contracting officer how much “cloud” he needed to buy, because not knowing is exactly the point of this technology. Jez was looking to acquire a cloud capability; contracting could only acquire a fixed, known quantity.
The contracting officer wanted to help Jez, but continued to insist that nothing could move forward without specifying a fixed amount. Jez explained the value of the cloud computing model in every way he knew how. It’s a bit like gas for your car, he tried, to no avail. They went back and forth for over two hours. Finally the contracting officer took a deep breath and said, “Let me explain how contracting works in the US government. We put in an order for 100 sandbags, we get 100 sandbags.” And the conversation was over.
Jez did ultimately succeed in buying cloud services (at terms far less favorable than the private sector because of government’s bespoke needs), but the process took orders of magnitude more effort, time, and money than it would have under a less rigid procurement framework. This rigidity has been a huge hindrance to the ability of government to serve its people; it will be even more obstructive if we hope to use AI. To ease that rigidity, we will need to provide agencies more flexibility, not less. We will need to enable more than we mandate.
Data ownership
Another example of how procurement will need to change is illustrated by our problems with data ownership. Processes for software acquisition over the past several years, grounded in misguided assumptions about how to evaluate vendors, have failed time and time again to ensure government’s access to its own data. Without data, there is no AI. The Office of Management and Budget’s (OMB) guidance to agencies about implementing the AI executive order stresses the need to treat data as a critical asset and ensure that contracts retain sufficient rights to data. This is essential moving forward, but there are few government agencies who can confidently say that they have those sufficient rights now, on both a legal and practical basis. (Sometimes, agencies seem to have the appropriate rights on paper, but when it comes to accessing data from their vendor, they find there are barriers, including but not limited to additional, unbudgeted costs.) This is particularly problematic when it comes to the equity audits that are now required for certain uses of AI by the new executive order.3. The majority of agencies now filing equity action plans lack the data needed to do so, some of which (but not all) is due to vendor control. In the meantime, for this reason and many others, adoption of AI to improve services will be stalled.
Once again, we have an issue that’s been problematic in the past, but becomes orders of magnitude more problematic in the AI era. Vendors will have even more powerful ways to stifle competition, lock agencies in, and skirt appropriate transparency and oversight unless government finally recognizes the value of its data and moves decisively to retain it. Vendors can be incredibly valuable partners in the mission, but the coming era requires government to step up and create the rules of the road for vendors to follow that truly serve the public.
Reducing burdens
Jez’s experience is also a great example of what I mean when I say that the other half of building capacity is reducing the burden on the people you have. Jez represents exactly the kind of talent we needed (and still need) in the Internet era: expert in the latest technologies, mission-driven, and a creative thinker. His counterparts in AI are the kind of people we seek to recruit today. And we succeeded in getting him to work in government for a time, between his tenure at hot start-ups and companies like Google. But he spent most of this time in government not deploying the latest technologies to improve government services, but fighting bureaucratic and administrative battles. The American people got some fraction of the value we could have had from Jez. We must not only recruit the right people, but do whatever we can to make it possible for them to do the job they came to do.
This imperative is not limited to tech workers. To improve customer experience, we will need far more people who understand data and technology. But what the public wants from customer service is answers: Where is my check? Why did I get this IRS notice? If we use AI just to make it easier to talk to government, but not to get those answers, we will fail. The key reason those answers are hard to come by is the enormous complexity of government programs. I worked on California’s unemployment insurance crisis during the pandemic, and encountered what is close to 10,000 pages of regulations governing what could be a relatively simple program. A claims processor working with my colleague kept calling himself “the new guy” because he was still learning the ropes. He had been with the agency for 17 years. But recall that unemployment insurance dates back to the 1935 Social Security Act. We’ve been adding rules and mandates for close to 90 years now. We almost never remove them. It’s no wonder the program is still in peril. It is collapsing under its own weight, weight that federal and state agencies can’t shed on their own.
It is tempting to say that AI will help us by understanding those 90 years of accumulated policy cruft for us. This is appealing in the short term and very dangerous in the long term. We can’t have a government so complex that one algorithm talks to the other at such a level of complexity that humans are out of the loop. Think about this problem in the context of the Department of Defense, where navigating the complexity and sheer volume of Pentagon policy — equivalent to 100 copies of “War and Peace” — slows everything from acquisitions to hiring to logistics to combat operations. I, for one, am not eager to live in a world where only AIs can tell our uniformed service members when they can and cannot shoot. But we can, and should, use AI to suggest dramatic simplifications to these overwrought frameworks and make those new leaner frameworks the law of the land. The greatest gift this body could give to the agencies and the American public they serve is a massive, thorough decluttering and spring cleaning. AI makes it possible, but only you in this chamber can make it happen.
Funding
Government procurement is a poor fit for competence in AI, but funding is upstream of procurement, and equally ill-suited to the task, in similar ways. Not only do we procure software as if it were static, we also fund it that way, and thus make it both worse and more expensive. This is best illustrated through a series of graphs, each one fictional but representative of two fundamentally different approaches to funding software (in both the current and coming paradigms.)
Government follows a “project” model. The following graph shows the number of staff who work on an IT project at its outset, as requirements are being developed, a request for proposal written, bids from contractors sourced and evaluated, and a winner chosen. The contractor, once hired, brings a team to develop the software based on the RFP, and the staffing levels (counting both internal and contracting staff) shoot up. There is a development period, followed by a short period of “user acceptance testing,” and then the project falls into “operations and maintenance,” which is generally a different “color of money” than the development funds.
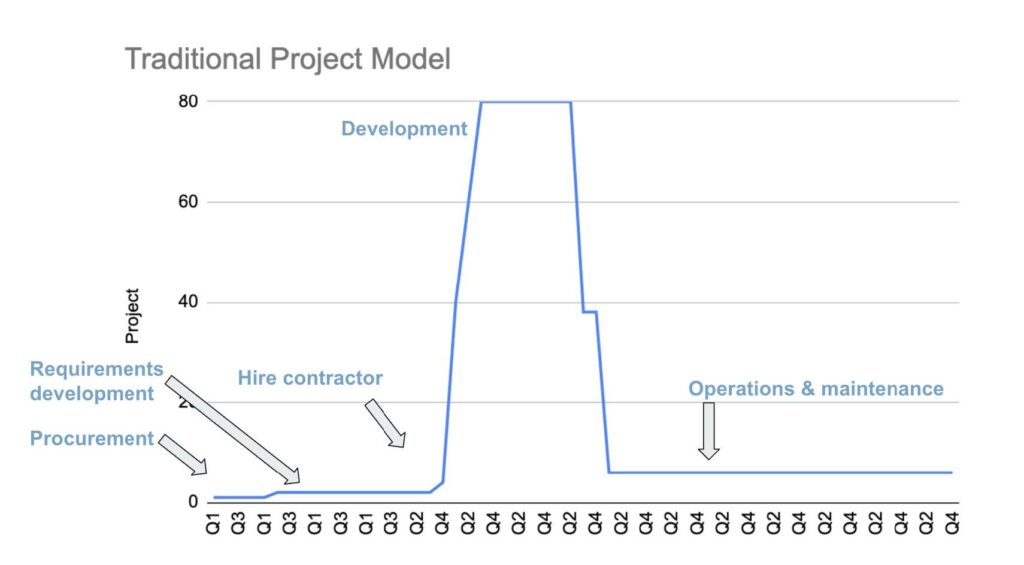
Contrast this with a typical “product” model, in which, instead of a requirements gathering phase up front, a small team, often but not always internal to government, conducts what are called discovery sprints to better understand the problems the software is supposed to address. If some parts of the proposed solution are riskier than others (for instance, it’s not clear whether a data integration will work well), they find ways to test those problems first, before an entire software solution has been built. They may develop prototypes to help question their assumptions, and they engage with users from the beginning. Product teams almost always leverage contractors, but the contractors are there to complement a core internal team which holds the product vision and provides clear direction to vendors. Staff is added slowly over time as the team learns what they need, but doesn’t dramatically ramp down once a first or even second version is shipped. As my colleague Dave Guarino quips, “Google didn’t lay everyone off after they put up search.” Indeed, they invested more.
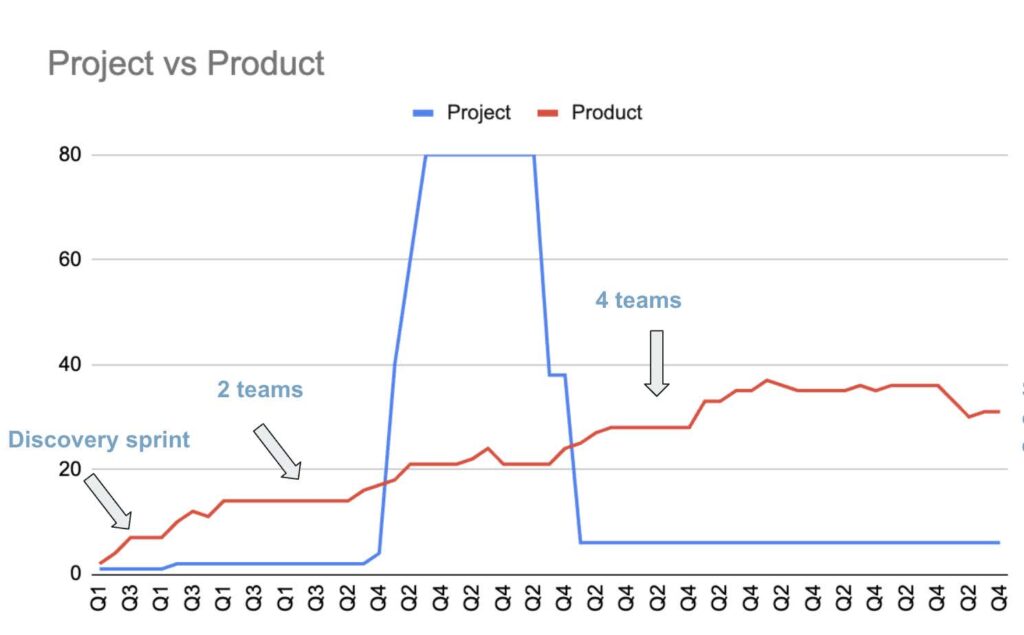
At this timescale, there seems to be an obvious reason to prefer the project model: the minimal ongoing expense. But as anyone following government technology appropriations knows, this is not the right timescale to look at. What happens next on the project line is one or more of the following scenarios: the software doesn’t work well for its users, and funds are sought to fix its defects; it quickly becomes outdated, either by changes in the technical environment, the policy environment, or other external factors, and funds are sought for modernization; or new needs have emerged that the existing software doesn’t address. Thus, the actual project model line looks more like this in the medium term:
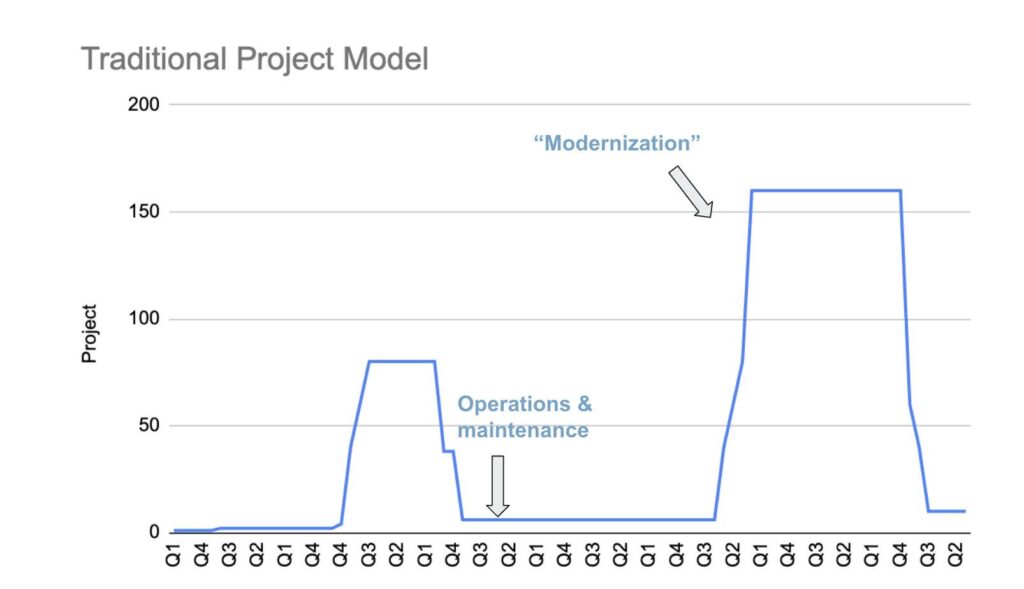
And then in the longer term, as modernizations fail, needs escalate, and even more money is allocated, like this:
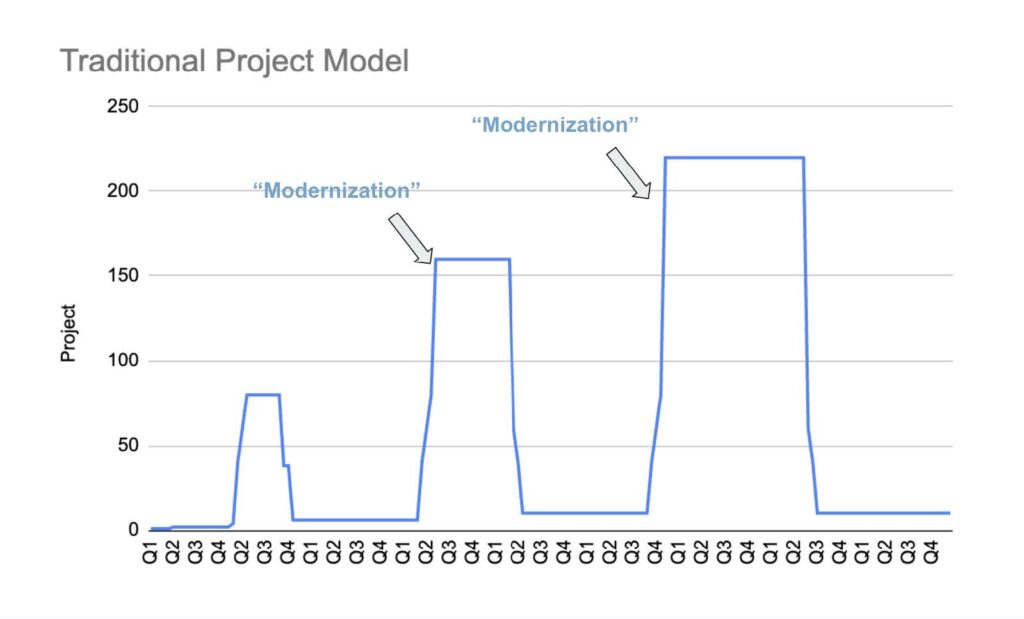
Here is where the slow and steady product line starts to look more attractive, on a purely cost basis, though cost is far from the only reason to prefer it. Having a consistent team over time may look like an unwanted ongoing expense, if we assume that development work at some point “is done,” but that is not the case. (“Software is never done” was one of the precepts of the Software Acquisition Practices report I contributed to for the Defense Innovation Board under President Trump).4 The product model is not only less expensive in the long run, it results in working software that rarely needs “modernization” of the kind you’ve become used to hearing about because it’s constantly being updated and improved. The biggest difference between the project and product models is that the steady investment over time delivers effective service to the American people. Periodic investment in “projects” is how we get backlogs and confused and frustrated constituents.
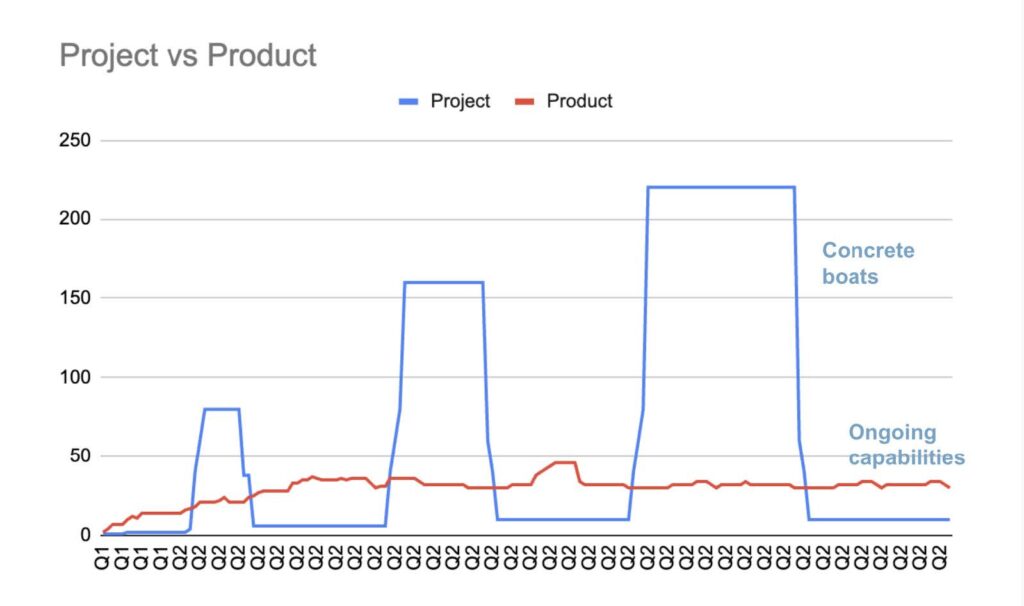
A summary of the differences in these models is below:
Project Model | Product Model |
Episodic large investments | Ongoing moderate investment |
Heavy reliance on procurement, oversight, and IV&V | Requires internal product ownership and management |
Abdication of responsibility to vendors | Partnership w/vendors |
Vendor lock-in | Low switching costs, smaller contracts |
Acquiring static software | Acquiring ongoing capabilities |
Constant loss of knowledge | Constantly growing understanding |
Customers consulted at end | Customers integral at all times |
Built in silos | The walls come down |
Subject to the “100% trap” | 85% to start costs 10% of the price |
High rates of failure and frustration | Actual working software |
As much as it’s tempting to hold agencies accountable for their addiction to the project model, this is not something they can fix on their own. Congress would need to enable ongoing funding streams (in addition to procurement changes previously discussed) in order to see agencies develop in the product model. Given all that we know about how fast AI moves, the risks it carries, and the benefits it could bring, Congress should work with OMB and agencies to change the laws, regulations, processes, and practices that impede agencies from operating in the product model.
Workforce
How will we get the AI workforce we need? OpenAI famously recruits talent with $1M signing bonuses. Government can’t compete on compensation, and it likely never will in this domain. But it competes remarkably well these days by selling the mission. For many in tech, the mission is irresistible. Organizations like the Tech Talent Project, which place digital professionals in roles in federal and state agencies, now have backlogs of tech leaders eager to serve the American public. For some, it was the pandemic’s brutal reminder of how much government matters. For others, it is threats to our country’s standing in the world that they want to counter. Whatever the reason, we now have people willing, and our greatest leverage will be in fixing the systems needed to actually hire them.
You would think that when we have proven tech talent ready to serve, we would jump to bring them on quickly. In fact, that backlog of tech leaders eager to join is largely languishing in hiring processes that can easily take nine months or longer. This could change, but it will require taking seriously the defects in our hiring practices. It’s not just speed, but how we hire. Today, 90% of competitive, open-to-the-public job announcements across the federal government rely solely on a resume review by an HR generalist and an applicant’s self-assessment of their skills.5 In other words, we have essentially one way to determine if candidates are qualified for the vast majority of positions — we ask them to rate themselves. Hiring managers often receive from HR staff a slate that contains no qualified candidates, which is why half of all hiring actions fail. They simply reject these slates and start over, adding even more months to what is already an unacceptable timeframe. Meanwhile, they miss qualified candidates whose resumes didn’t make the cut because they didn’t know the absurd games applicants must play to get placed on the HR hiring slates, like copying and pasting the qualifications noted in the job description directly into their resume, and rating themselves “master” at every single competency listed in the assessment. We are losing too many willing digital professionals, not because of lower pay, but because of arcane, cumbersome processes. Lack of flexibilities like remote work makes the problem even worse.
The Office of Personnel Management (OPM) and the White House have stated their intentions to hire the AI tech talent needed, but this is a case where strengthening the workforce is also a matter of reducing burdens. OPM’s recent memo, for instance, will grant direct hire authority for several AI-related job classifications. That will remove a bit of the red tape agencies need to bring on experts. But that direct hire authority does not allow for the use of pooled hiring across agencies, despite the fact that pooled hiring has gotten us many excellent data scientists and other tech roles much more quickly. Agencies will have to run a separate hiring action for each open position, which will take enormous amounts of time and paperwork, even with the direct hire authority. Congress should ask OPM what authorities they need in order to change this, and what resources they need to scale programs like the highly successful Subject Matter Expert Qualifying Assessment (SME-QA) program. Then ask what is the next obstacle they need removed. I don’t presume to know everything that is needed, only that they operate in a highly constrained environment, no longer fit to the purpose it must serve.
For those who despair of our ability to compete for talent, it’s important to remember that the people OpenAI and others are hiring at such sky high salaries are typically those who know how to develop and train models. Government’s primary need is not for that very specialized talent pool. It is for people who know how to use these models. Though I am a fan of the notion of government creating its own models, that will be the extremely rare exception. The commercial and open source communities will provide models government can adopt. The expertise needed to take advantage of AI software developed by others is at far less of a premium than that of the talent pool getting the $1M signing bonuses, and it is even more critical to successful adoption. The kinds of technologists that USDS, 18F, and federal agencies have been hiring and continue to hire – service designers, product managers, data engineers – can do this work, even if they are not technically experts in AI (though some are). We just need to hire them at a much greater scale.
Greater competence and capacity are also important because we need people who use AI, when appropriate, to solve real problems. There is the very real risk that agencies, especially those that lack sufficient basic digital expertise, will buy AI tools in ways that are compliant with all the new guidance, but that fundamentally lack an understanding of the problem they are trying to solve. We’ve seen this many times before in government and elsewhere, especially with blockchain technologies – a rush to sprinkle “advanced tech fairy dust” on a tech portfolio without a clear purpose or a clear match between the need and the solution. These thoughtless implementations will harm the public, give AI in customer service a bad name, and understandably strengthen the calls to slow down. The more uses of AI for AI’s sake, the more we risk stifling what could be a welcome advancement if done thoughtfully.
AI can’t be done thoughtfully without the right workforce. And we can’t legislate our way to the right workforce, though removing previous legislative mandates may help. Congress will need to encourage and enable OPM to build the human resources system we need to meet this moment.
An enablement approach
It can be difficult to legislate competence in digital or any other domain. A large part of what makes us bad at customer experience in a digital age is that we have created a system in which the careers of government staff depend more on compliance with process than on achieving the desired outcomes. More rules usually exacerbate this effect, leading, ironically, to worse outcomes. Even legislation that doesn’t add rules, but simply directs the executive branch to make studies or plans can lead to more unhelpful rigidity.
In my book Recoding America I tell a story of a team unable to ship the software for the new GPS satellites because they’ve been told that a certain component, one that breaks the software, is required by law. Many people up and down the hierarchy literally believe that Congress has mandated this component. Because of this belief, no one can get approval to take it out, even though the software has gone years over schedule and billions of dollars over budget, and would finally work if this component were removed. (It never was.)
Congress, of course, had not mandated that this specific component be used in this specific software project. In the 1996 Clinger Cohen Act, Congress had mandated that OMB provide high level guidance around interoperability in software, and this component was used to illustrate how interoperability might be achieved. As that high level guidance was translated into ever more concrete and binding policies at lower levels of within government, risk aversion caused it to go from an illustration to a recommendation to a binding requirement, in this case dooming the project. Even when legislation is written with sufficient leeway to allow implementers to use their judgment, it runs the risk of causing the sort of calcification that leads to bad outcomes. We must be careful what we legislate lest it have negative unintended consequences.
The goal, therefore, must be Congressional action that reduces the risk aversion of the bureaucracy. Simplification of accumulated policy cruft as described above (with help from AI) falls into this category. Careful use of oversight, including to lift up successes as often as we question failures, counts as well. Getting government agencies the people they need, focused on the right work, and reducing the burden on each of them, can be profoundly transformational.
Conclusion
As we enter the AI era, we are forced to finally grapple with the lessons of the Internet era. Chief among those lessons is how much lack of digital capacity in government has hurt the American people. Fortunately, we already know much of what we need to do to face this challenge, because it is largely the same work we have needed to do for the past two decades. AI is still software, just software that intensifies and speeds up the need for change that we’ve observed to date. Its arrival is our wakeup call to do what we should have already done, but it is also a gift that will help us do this work.
Notes
- Why the American People Deserve a Digital Government, Clare Martorana, Federal CIO,
September 22, 2023
https://www.whitehouse.gov/omb/briefing-room/2023/09/22/why-the-american-people-deserve-a
-digital-government/ ↩︎ - Wu, Eric, Kevin Wu, Roxana Daneshjou, David Ouyang, Daniel E. Ho, and James Zou. 2021. “How Medical AI Devices Are Evaluated: Limitations and Recommendations from an Analysis of FDA Approvals.” Nature Medicine 27 (4): 582–84. ↩︎
- Gupta, Arushi, Victor Wu, Helen Webley-Brown, Jennifer King, and Daniel E. Ho. 2023. “The Privacy-Bias Tradeoff: Data Minimization and Racial Disparity Assessments in US Government.” In Proceedings of the 2023 ACM Conference on Fairness, Accountability, and Transparency, 492–505. ↩︎
- https://innovation.defense.gov/software/ ↩︎
- 5GSA’s Hiring Assessment and Selection Outcome Dashboard
https://d2d.gsa.gov/report/hiring-assessment-and-selection-outcome-dashboard ↩︎